Artificial Intelligence Must Be More Responsible Than Humans
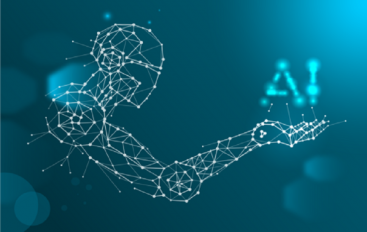
Since the dawn of Bronze age civilizations more than 5000 years ago, humans have been creating norms of societal governance. The process continues with many imperfections. Off late, Artificial Intelligence (AI) is increasing its influence in decision making processes in the lives of humans and expectations are whether AI will follow similar or better norms. Principles that govern the behaviour of responsible AI systems are being established.
Principles
Fair
All AI systems should be fair in dealing with people and be inclusive in coverage. In particular, they should not show any bias in working. Historically, humans have used at least 2 major criteria for unfair treatment, i.e. gender and caste/race/ethnicity.
Amazon tried to develop an algorithm for recruitment. However, it started showing less tendency to select female candidates. Even after removing gender specific indicators, females were still discriminated against. The project had to be abandoned.
Compas, a risk-assessment tool developed by a privately held company and used by the Wisconsin Department of Corrections predicted that people of colour had higher tendency of repeat offences than they actually do. California has decided not to use face recognition technology for law enforcement. A study by Stanford researchers in 2020 found that voice recognition software of Amazon, Apple, Google, IBM, and Microsoft have higher error rates when working on voice of black people.
Transparent and Accountable
Unlike traditional software, it is hard to predict the outcome of AI algorithms as they dynamically change with training. This makes them less transparent and this “Black box” nature of AI makes it very difficult to find the source of error in case of a wrong prediction. This also makes it makes difficult to pinpoint accountability. Neural networks are the underlying technology for many face, voice, character etc recognition systems. Unfortunately, it is more difficult to trace problems in neural networks especially deep ones (with many layers) than in other AI algorithms e.g decision trees etc. And new variants of neural networks e.g. GANs (Generational Adversarial Networks), Spiking Neural Networks etc continue to gain popularity.
Reliable and safe
Security and reliability of AI systems has certain peculiar dimensions e.g. unpredictability. Facebook in collaboration with Georgia Institute of Technology created bots that could negotiate but they also learnt how to lie. This was not intended during programming. Another issue is slow rise of Artificial General Intelligence (AGI) or Broad AI or Strong AI that aims to create systems that genuinely simulate human reasoning and generalize across a broad range of circumstances. These algorithms will be able to do transfer learning, so an algorithm that learns to play Chess will also be to able learn how to play Go. This will vastly increase the context in which a machine can operate and this cannot be predicted in advance.
Unpredictability reduces reliability and safety of the systems.
Problem sources
Models and features
The power of AI algorithms is based on the models and features and the weightages of the features that are used while creating models. The AI in use currently is also Narrow AI and it will not work if the context changes. For example, a system designed to scrutinize applications for medical insurance policies may discriminate against people with diseases if used to vet applications for car insurance since the features and their weightages are not appropriate for the latter case. Hence models or features framed without fairness in mind can induce biases.
Data
The biggest source of biases in AI systems is data as biases may be inherent in the data, either explicitly or subconsciously. This can happen if data is not uniformly sampled or carries implicit historical or societal biases. In credit risk, data of customers who defaulted less as they were supported by tax benefits will give incorrect results when used for scenarios where tax benefits are not there. MIT researchers found that facial analysis technologies had higher error rates for minorities and particularly minority women, potentially due to unrepresentative training data. The reason for failure for Amazon recruitment software was that it was trained on 10 years of data where resumes of male candidates outnumbered that of females. It also focussed on words e.g. “executed”, “captured” etc that are more commonly used by males.
Other issues
The rise of AI poses additional challenges not found in traditional systems.
Driverless Vehicles
The driverless vehicles will start plying on the roads in a decade or so. Any accident will raise the question of civil and criminal liability. In 2018 a pedestrian died when she was hit by Uber test car despite a human driver sitting inside the car. A vehicle may be programmed to save either the passengers or pedestrians. Potential accused could be vehicle manufacturer, vehicle operator or even the government. This will also change the underwriting models. Liability issues will also come as companies allow operation decisions to be more data driven as now programmers will appear to be the sole accused.
Weapons
Countries e.g. US, Russia, Korea etc plan to use AI in weapons e.g. drones or robots etc. Currently the machines do not have emotions and this raises the concern if an autonomous machine goes on killing spree. In 2018, Google had to stop engagement with US government over its Maven military program due to public outcry.
Safeguards
Guidelines
The concerns over ethics in AI have resulted in many organizations formulating guidelines governing the use of AI e.g. European Commission's, "Ethics Guidelines for Trustworthy Artificial Intelligence", US government’s “Roadmap for AI Policy”, IEEE’s P7000 standards projects etc. These contain the general principles of ethics and responsibility that AI systems should follow.
Software
Many companies have created frameworks, software, guidelines etc that can help to create Responsible AI e.g. IBM, Google, Microsoft, PWC, Amazon, Pega, Arthur, H2O etc. Their software help to explain model’s “Black box” behaviour and hence bring transparency, assess fairness of the systems, mitigate bias against any identity based groups, keep the data secure etc by constant monitoring.
Companies
Within companies, Responsible AI can be facilitated by imposing standards through overseeing groups, creating diversity in teams and cascading the message to individuals. There should be conscious efforts to reduce biases in data.
Future
In the next two decades, machines will become more autonomous in decision making processes and human will slowly cede control of their own lives. Establishment of Responsible AI will reduce biases and increase acceptance of AI. This will help in creating a more fair and equitable society. An unchecked growth of AI will not only make humans less tolerant to AI but also to each other.